Model-Data Integration
Dr. Nuno Carvalhais
Mission
In general, we are curious about interactions in carbon and water dynamics in terrestrial ecosystems in an Earth system context, and are keen on learning by bringing together models and observations. We want to advance understanding in interactions between terrestrial ecosystems and climate with a particular relevance to the global carbon and water biogeochemical cycles. The MDI group explores strategies and develops methods to extract and transfer information from data to models to investigate the functioning --dynamics and sensitivities-- of terrestrial ecosystems and its representation in modeling structures. Ultimately, we aim at identifying approaches that are robust against inevitable challenges in data and models to represent complex Earth system processes.
Focus areas
Model-data-integration : Exploration of multiple-constraint model-data assimilation approaches using in situ and Earth Observation data-streams of water and carbon states and fluxes
We have been investigating in situ dynamics of carbon and water fluxes for parameter inversion, for model selection and for understanding parameter variability; and, at global scales, to investigate controls on trends in [CO2]atm and evaluate contrasting descriptions of large scale hydrological dynamics. The focus on "multiple-constraints" approaches and combining classical inverse parameter estimation techniques with machine learning approaches paves way to SINDBAD framework (see below), and the development of hybrid modeling approaches therein. We are very interested in methodological aspects of breeding model-data-assimilation and machine learning approaches to maximize the information flow from observations to models.
Terrestrial C-H2O dynamics across scales : Exploration of observation-driven approaches to diagnose (1) determinants of carbon sink/source strength and (2) carbon-water coupling strength at ecosystem and watershed scales.
Based on statistical approaches, we study carbon sink/source strength for the FLUXNET domain, and investigate, in addition to climate, the importance of stand age and biomass dynamics and distribution. The approach paves way for improvements in observation-based upscaling of NEP fluxes. In a strong collaboration with GDM, we support the development of observational-based approaches to diagnose physiological responses to water stress and the use of different approaches to partition surface-atmosphere water fluxes.
Ecosystem carbon turnover times: Diagnosing and interpreting mechanisms underlying the environmental controls on ecosystem turnover times of carbon.
We investigate carbon turnover times as an observational diagnostic on the strength of the land-atmosphere carbon cycle coupling. We have been working on breaking down an whole ecosystem estimate into different components (vegetation and soil), revisiting the importance of scale and equilibrium assumption to investigate the robust features in the observation-derived turnover times. We are interested in observational and methodological aspects to understand biotic and abiotic controls on temporal dynamics of carbon turnover; in developing further concepts to constraint projections from Earth System Models; in contributing to observational dataset development
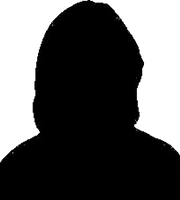
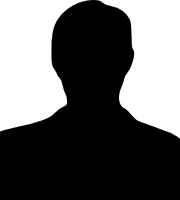
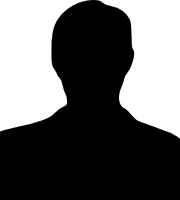
Other members of the department actively involved in MDI activities
Guests
Yang, H.; Wang, S.; Son, R.; Lee, H. T.; Benson, V.; Zhang, W.; Zhang, Y.; Zhang, Y.; Kattge, J.; Boenisch, G. et al.: Global patterns of tree wood density. Global Change Biology 30 (3), e17224 (2024)
Son, R.; Stacke, T.; Gayler, V.; Nabel, J. E. M. S.; Schnur, R.; Alonso, L.; Requena Mesa, C.; Winkler, A.; Hantson, S.; Zaehle, S.; Weber, U.; Carvalhais, N.: Integration of a deep-learning-based fire model into a global land surface model. Journal of Advances in Modeling Earth Systems 16 (1), e2023MS003710 (2024) doi. org/10.1029/2023MS003710
Bao, S.; Alonso, L.; Wang, S.; Gensheimer, J.; De, R.; Carvalhais, N.: Toward robust parameterizations in ecosystem‐level photosynthesis models. Journal of Advances in Modeling Earth Systems 15 (8), e2022MS003464 (2023), doi.org/10.1029/2022MS003464
Yang, H.; Munson, S. M.; Huntingford, C.; Carvalhais, N.; Knapp, A. K.; Li, X.; Peñuelas, J.; Zscheischler, J.; Chen, A.: The detection and attribution of extreme reductions in vegetation growth across the global land surface. Global Change Biology 29 (8), pp. 2351 - 2362 (2023) doi.org/10.1111/gcb.16595
Fan, N.; Reichstein, M.; Koirala, S.; Ahrens, B.; Mahecha , M. D.; Carvalhais, N.: Global apparent temperature sensitivity of terrestrial carbon turnover modulated by hydrometeorological factors. Nature Geoscience 15, pp. 989 - 994 (2022) doi.org/10.1038/s41561-022-01074-2
Besnard, S.; Santoro, M.; Cartus, O.; Fan, N.; Linscheid, N.; Nair, R.; Weber, U.; Koirala, S.; Carvalhais, N.: Global sensitivities of forest carbon changes to environmental conditions. Global Change Biology 27 (4), pp. 6467 - 6483 (2021) doi.org/10.1111/gcb.15877
Analysis of climate constraints on gross primary productivity based on light use efficiency models : Here, Shanning analyzes the patterns of climate sensitivities of gross primary productivity using FLUXNET data and global sun-induced fluorescence data. The project focuses on the evaluation and prediction of climate sensitivity functions and model parameters based on model-data fusion strategy.
Ecological process understanding across time scales: In her PhD project, Nora explores time-scale dependencies between vegetation and climate globally, to answer questions such as: How does the decadal sensitivity of vegetation to climate differ from seasonal sensitivity? Can we extrapolate atmosphere-biosphere relations from one time scale to the other? Where and when do we need to account for such time-scale dependencies in vegetation modeling and monitoring?
Water-carbon-cycle interactions across scales : In his PhD project, Hoontaek attempts to answer 1) how interactions between water and carbon cycles form observations of each cycle and 2) who drives whom at which scales. To do so, Hoontaek will take advantage of the stream of global datasets and a model-data-fusion framework.
Change in global vegetation : Here Siyuan explores data-assimilation to study vegetation processes and ecosystem responses to environment at different spatio-temporal scales. The project investigates the links between ecosystem carbon fluxes and changes in vegetation carbon states, integrating vegetation mortality and metabolic activity based on a novel modular modeling framework and different observational data streams.
Inter-annual variability in terrestrial ecosystem fluxes, in this PhD project, Ranit endeavours to develop a hybrid modelling approach to simulate water and carbon fluxes at the ecosystem level. The project will exploit large multi-variate Earth Observation datasets to explore causal linkages between changing climate and ecosystem responses by taking advantage of both physically based models (physically interpretable) and machine learning approaches (data-adaptive)
Alumni
Carbon turnover times: Naixin has synthesized and processed multiple global datasets of gross primary productivity, soil carbon, and vegetation carbon, to generate a large ensemble of global whole ecosystem carbon turnover times (Fan et al., 2020). The previous dataset developed by Nuno has already been used for evaluation of Earth System Models (Carvalhais et al., 2014). Both these data are publicly available through the data portal of Max Planck Institute for Biogeochemistry.
ESMValTool: The Earth System Model Evaluation Tool (ESMValTool) is a community-development that aims at improving, diagnosing and understanding the causes and effects of model biases and inter-model spread. Within CRESCENDO project, Sujan contributed an ESMValTool “recipe” that diagnoses the ESM simulations of 𝝉.
Forest age: based on forest inventory data, Simon developed a ML model to derive forest stand age from global remote sensing and climate data.
Global Aboveground Biomass: we collaborated with GAMMA Remote Sensing, within the GlobBiomass ESA DUE consortium, to develop a global high resolution map of above ground biomass. The data can be found here: https://doi.pangaea.de/10.1594/PANGAEA.894711.
Lambda: Çağlar (GDM/MDI) developed a new ecohydrological dataset of metrics characterizing the vegetation dynamics under moisture limitation in Africa using daily time series of Fraction of Vegetation Cover (FVC) from the geostationary METEOSAT satellite (Küçük et al., 2020).
TEA: Transpiration Estimation Algorithm: Jake (GDM/MDI) makes available data (https://zenodo.org/record/3978408#.YECpgWLPx_V), the algorithms and some of the tools developed in investigating the evapotranspiration flux partitioning from eddy covariance data (https://github.com/jnelson18/).
Over the years we have been hosting internships, students working on their Masters thesis, and mostly the development of PhD and Postdoc research projects. These have been opportunities for training and for the development of collaborative research that have been mutually appreciated, and where I have seen that we have grown as a group. If you are interested in working with us, feel free to get in touch. We announce opportunities here and in the context of the IMPRS for PhD projects.